Community
Audio and visual resources created by and for people interested in addiction
Podcasts
Publishing Addiction Science
A podcast for addiction scientists facing the complex process of academic publishing.
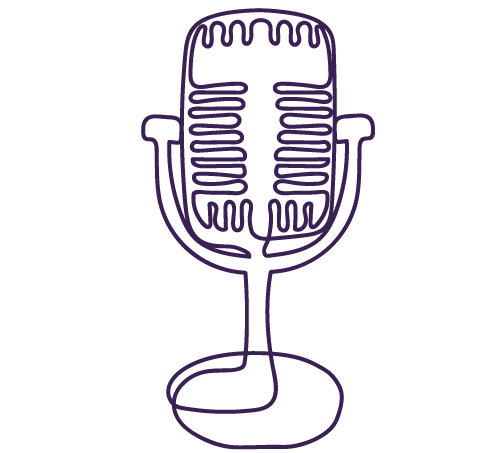
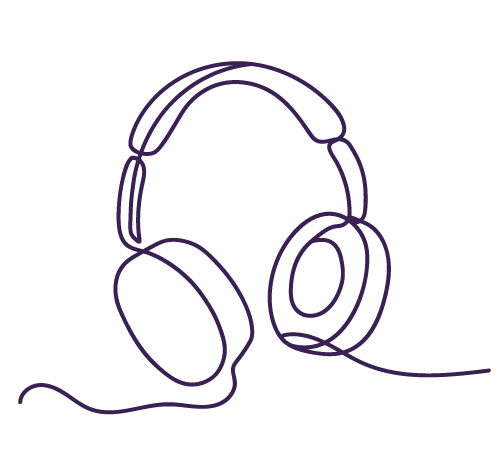
Addiction Audio
The podcast from Addiction journal, featuring interviews with authors about their research.
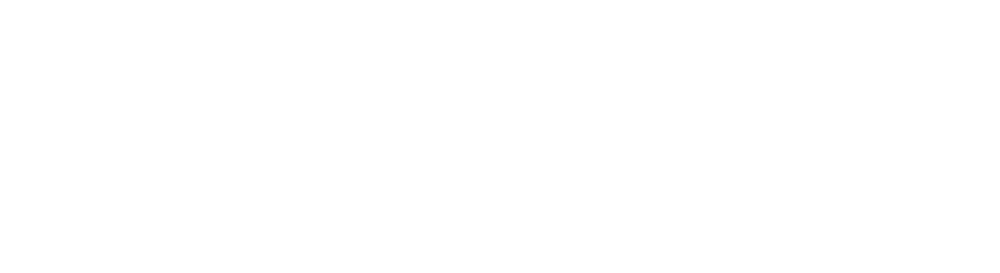
Interviews
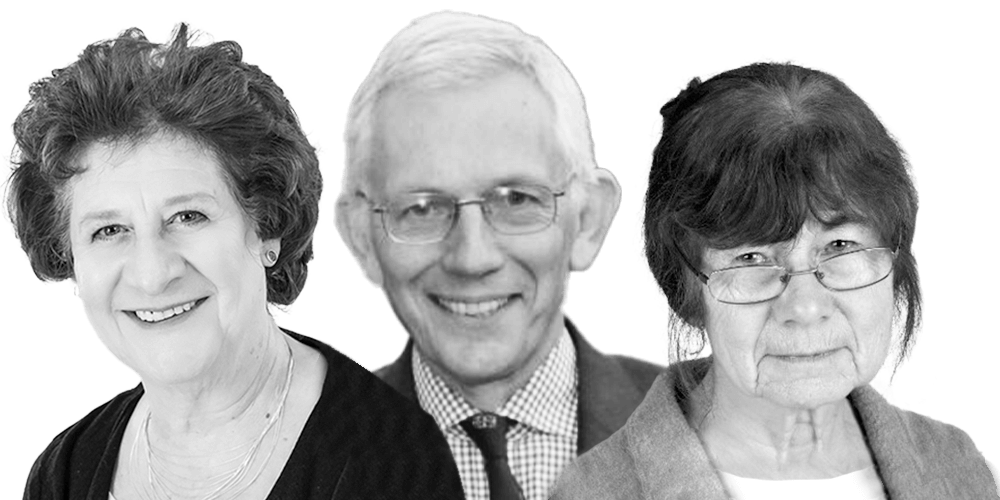
Addiction Lives
An interview series preserving the memories and experiences of people who work in the field of addiction.
Women in Addiction
The Women in Addiction project is a series of short films highlighting women who have made a contribution to the field of addiction.
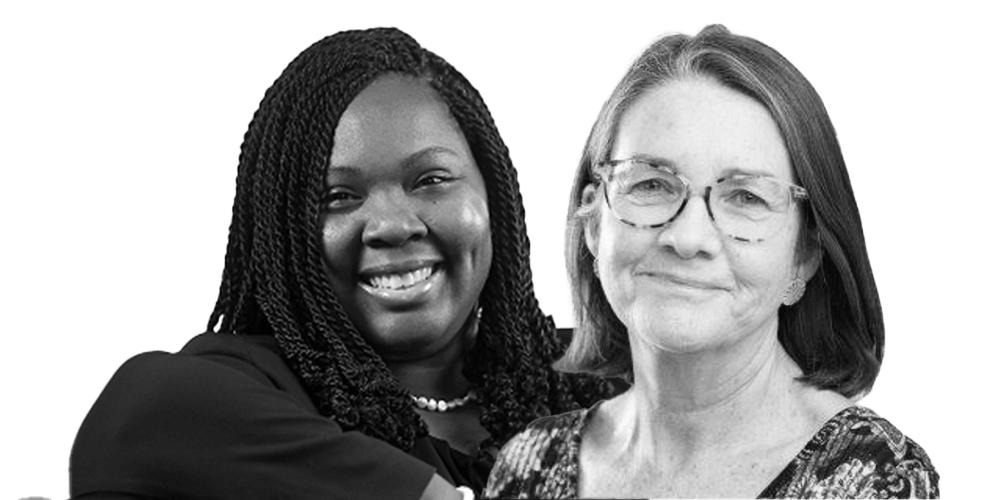